Keynote Speakers
Toward 3D Visual Perception and Trustworthy Biometrics
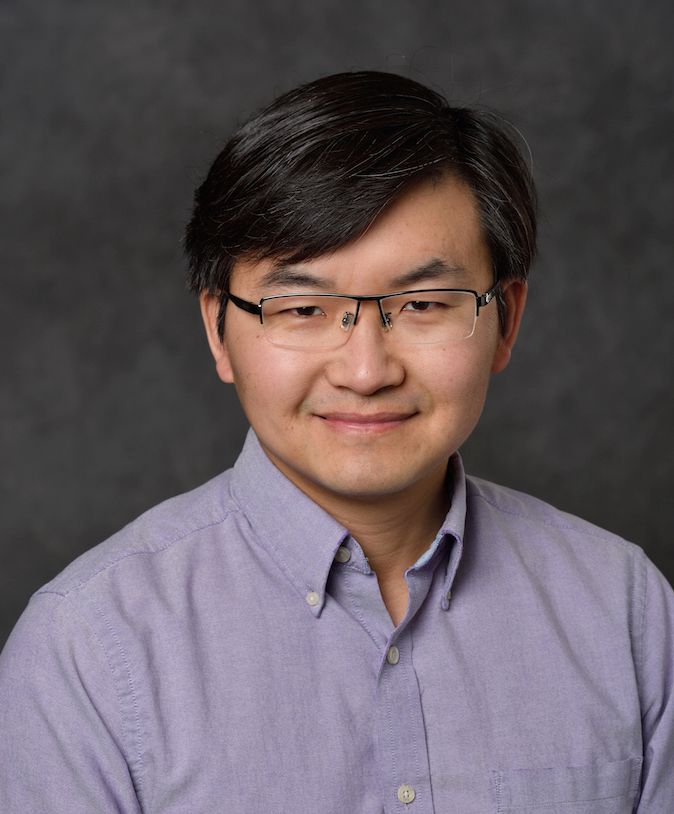
Dr. Xiaoming Liu
MSU Foundation Professor Department of Computer Science and Engineering - Michigan State University
Abstract
Visual perception of the scene and identification of individuals are conventional tasks of the AVSS community. In the deep learning era, many new research thrusts are being developed for these conventional tasks. In this talk, we will share two thrusts based on the research conducted at the Computer Vision Lab at Michigan State University. The first is the 3D perception, i.e., detection, tracking and forecasting moving objects in the 3D space. While most of existing 3D perception algorithms rely on depth sensors such as LiDAR, we will start with our monocular 3D perception works, and then discuss the new sensing capability enabled when fusing with additional radar or LiDAR sensors. The second is trustworthy biometrics. In recent years we have witnessed increasing application scenarios of biometrics systems in our daily life, despite the societal concerns on some of the weakness of the technology. To address these concerns, trustworthy biometrics has become an emerging research thrust. Specifically, we will discuss various topics such as biometrics security (e.g., presentation attack detection and forgery detection), biasness in biometrics, adversarial robustness, and interpretable biometrics.
Biography
Dr. Xiaoming Liu is the MSU Foundation Professor at the Department of Computer Science and Engineering of Michigan State University (MSU) and also a visiting research scientist at Google Research. He received Ph.D. degree from Carnegie Mellon University in 2004. Before joining MSU in 2012 he was a research scientist at General Electric (GE) Global Research. He works on computer vision, machine learning, and biometrics especially on 3D vision, and face related analysis. Since 2012 he helps to develop a strong computer vision area in MSU who is ranked top 15 in US according to the 5-year statistics at csrankings.org. He received the 2018 Withrow Distinguished Scholar Award from MSU. He has been Area Chairs for numerous conferences, including CVPR, ICCV, ECCV, ICLR, NeurIPS, ICML, the Co-Program Chair of BTAS’18, WACV’18, IJCB’22 and AVSS’22 conferences, and Co-General Chair of FG’23 conference. He is an Associate Editor of Pattern Recognition and IEEE Transaction on Image Processing. He has authored more than 160 scientific publications, and has filed 29 U.S. patents. His work has been cited over 15000 times according to Google Scholar, with an H-index of 60. He is a fellow of International Association for Pattern Recognition (IAPR). His research has been widely reported in prominent national and international news outlets including the Wall Street Journal, CNBC, CNET, Engadget, Fortune, the Mac Observer, MSU Today, New Scientist, Silicon Angle, VentureBeat, and the Verge. More information of Dr. Liu’s research can be found at http://cvlab.cse.msu.edu
Learning from Small Data and Without Labels
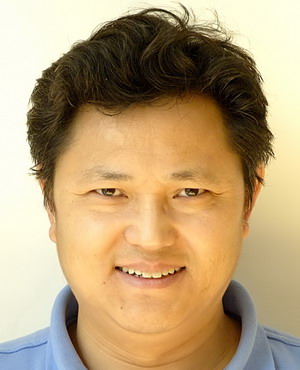
Dr. Shaogang Gong
School of Electronic Engineering and Computer Science - Queen Mary University of London
Abstract
Deep learning has flourished in computer vision and AI over the past decade. This is due in no small part to the availability of centralised big training data with extensive labelling. However, increasing awareness of privacy concerns and a renewed focus on decentralised user-ownership of localised data poses new challenges to the conventional wisdom for centralised deep learning on big data, especially for applications in human recognition tasks such as person re-identification. Moreover, Convolutional Neural Networks are mostly designed for supervised learning from labels therefore become significantly weaker when there is no label in unsupervised learning. In this talk, I will discuss challenges and progress on federated learning from decentralised independent label domains and semantic contrastive learning for self-supervised deep clustering with applications in generalising person re-identification to novel domains without training and unsupervised learning image search.
Biography
Dr. Shaogang Gong is Professor of Visual Computation at Queen Mary University of London since 2001 (http://www.eecs.qmul.ac.uk/~sgg/) and the Director of the Computer Vision Group. He is a Turing Fellow of the Alan Turing Institute – the UK's national institute for data science and artificial intelligence, a Fellow of ELLIS – European Laboratory for Learning and Intelligent Systems, a Fellow of IET, a Fellow of BCS, and a Member of the UK Computing Research Committee – the Expert Panel of the British Computer Society, the UK Council of Professors and Heads of Computing, and the Institution of Engineering & Technology. He served on the Steering Panel of the UK Government Chief Scientific Adviser’s Science Review. He has authored and edited 7 books on Person Re- Identification, Visual Analysis of Behaviour, Video Analytics for Business Intelligence, Dynamic Vision from Images to Face Recognition, Analysis and Modelling of Faces and Gestures. He has made unique contributions to the engineering of CCTV video analytics recognised by the law enforcement and the security industry. He was awarded the Institution of Engineering & Technology 2020 Achievement Medal for Vision Engineering for “outstanding achievement and superior performance in contributing to public safety”. A software system developed based on his research won the 2017 Global Frost & Sullivan Award for Technical Innovation on Law Enforcement Video Forensics Technology, and the 2017 Aerospace Defence Security Innovation Award for “revolutionary solution to reviewing CCTV footage”. He founded the Queen Mary Computer Vision Laboratory in 1993, and received his DPhil in computer vision from Keble College, Oxford University in 1989.